AI tools come with risks. This Wharton professor is teaching ‘accountable AI.’
University of Pennsylvania’s Wharton School professor Kevin Werbach hosts the podcast "The Road to Accountable AI."
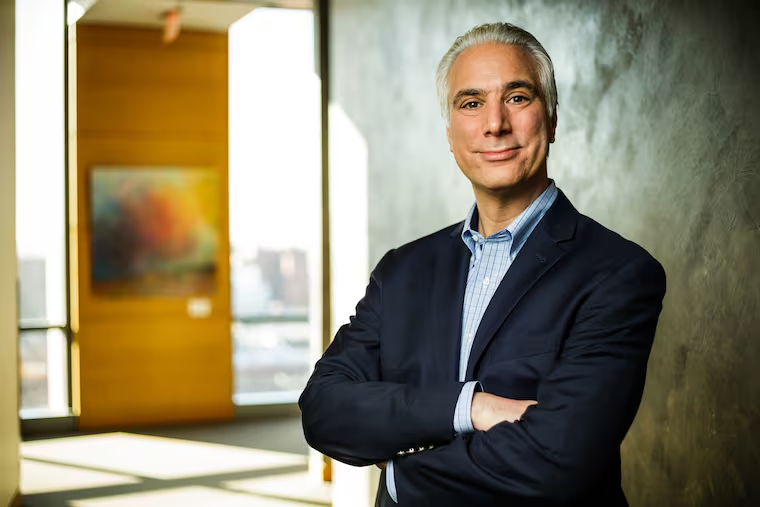
Businesses are already using AI, but how are they going about addressing their risks?
This fall, Kevin Werbach, professor of legal studies and business ethics at the Wharton School of the University of Pennsylvania, is leading a new course as part of the school’s executive education program that will focus on “accountable AI,” a term he uses to refer to the practice of understanding and addressing AI risks and limitations.
Some 18% of S&P 500 companies mention AI in their 2023 annual reports as a “risk factor,” Werbach explains on the first episode of his podcast, The Road to Accountable AI, which launched in April.
“That probably should have been higher,” he says on the podcast.
In an interview with The Inquirer, Werbach explains some AI issues and what accountable AI entails.
“There have been example after example of failures of AI systems, systems that are biased based on race and gender and other kinds of characteristics, systems that make very significant errors,” he said. “The ordinary person out there who is hearing about AI is probably hearing at least as much about the failures as the benefits today. The reality is both are real, but to me that means we need to think about how to maximize the benefits and minimize the dangers.”
The interview has been condensed for brevity and clarity.
What is accountable AI?
Accountable AI is the phrase that I prefer to describe the practice around understanding and addressing the various kinds of risks and limitations of implementation of AI. People sometimes talk about AI ethics, which is a piece of it, but there’s a danger that just focusing on ethics leads people to emphasize the principles as opposed to practical steps to take in organizations. Sometimes there’s talk about responsible AI — which is closer — but still doesn’t necessarily get organizations focused on how to create effective structures of accountability. So I talk about accountable AI as the entire set of frameworks around understanding issues with AI systems, figuring out how to manage them, govern them, mitigate risks, and putting into place mechanisms of accountability so people feel that they are the ones who have the obligation to take the kinds of actions that are necessary.
In your podcast you mention a Pew 2023 survey in which a majority of respondents were more concerned than excited about AI in daily life. Why are you excited about AI, and do you think others should be, too?
I’m excited about AI because there are countless ways that we can use it either to do things humans are doing — better or faster — or in some cases to do things at scales that humans really can’t effectively do. Especially with the rise of generative AI, where this is not just machine learning that gets developed and implemented by data scientists, it’s something anyone in an organization can interact with directly. There’s just an infinite number of places where we’re going to find out ways that AI will make business more effective and potentially make people’s lives better.
On the other hand, there are huge, huge problems and concerns. Those range from small-scale issues — we know that large language models (generative AI systems like ChatGPT) will hallucinate and create information that’s just simply wrong — to there could be catastrophic effects of these technologies being used for weapons development and terrorism and so forth, and everything in between.
What does regulation for AI look like today? Should businesses be creating their own internal policies?
Definitely businesses should be creating their own internal policies. There are a whole range of different issues. ... So for example, if you do not have an enterprise license to these tools, then typically any queries that you send to the chatbot get stored and can be used by the company that’s providing that service. If you’re in a financial services firm and someone is asking a question that reveals very sensitive, confidential information for the firm, you might just think “it’s like I’m typing in a search term to Google,” but potentially, you’re giving up sensitive private information, which could then be used to train future models and [be] accessible to the rest of the world … that’s something an organization should think about.
What are some of the ethical considerations business should be thinking about when choosing to implement AI tools into their processes?
It’s really important for businesses to think about what are their general ethical principles that are important to them. That’s something that they probably should be doing already with technology. We’ve had many years of controversies about issues like privacy and security and fairness — those are ethical values that are relevant to technology in general, which are very relevant to AI. … If a health-care firm is using AI to read X-rays effectively, that’s something different from a marketing firm that’s using AI to generate copy, but there are ethical issues in both contexts. It’s really a matter of mapping out the major ethical issues that the firm’s concerned with.
Do you think more businesses should be using AI? Is this a good moment for businesses to be trying this out?
Everyone should at least understand what the technology is and what it’s capable of. The generation of generative AI systems — the chatbots and so forth — are so novel and powerful in ways that we haven’t really experienced before, that everyone should at least get a handle on them. It doesn’t necessarily mean everyone needs to adopt them or they’re going to change everyone’s life overnight, but everyone should understand what they do well and don’t do well, and how far along we are, and how fast the technology is evolving, because otherwise they’re going to be surprised. This is accessible to everyone, so your competitor very well may be experimenting with the technology if you’re not.
What are some of the risks of using AI tools, and how can businesses protect themselves or mitigate them?
One risk is accuracy, especially with generative AI. One big challenge is you may not quite understand why the AI produced a certain result, which in some situations might not be important, but in a situation where, let’s say the AI system says hire this person and not that person, and the person who didn’t get hired challenges that decision, how do you explain that “the AI told me to”? There’s a big set of technical challenges around explaining AI.